Aradillas-López and Tamer (2008)
These slides are based on the July 2007 version of the following article:
Aradillas-López, Andrés, Andres and Elie Tamer (2008). The Identification Power of Equilibrium in Games. Journal of Business and Economic Statistics 26, 261–283.
Presentation by Jason Blevins, Duke Applied Microeconometrics Reading Group, December 4, 2007.
Introduction
Examines the identification power of Nash equilibrium assumptions.
Compare results by dropping Nash equilibrium and using only rationalizability.
Three examples are considered:
2x2 game of complete information (e.g., Bresnahan and Reiss (1991)),
2x2 game of incomplete information,
First price auction with independent private values.
Given a random sample, what can we learn about a parameter of interest using only level- rationalizability.
Equilibrium Concepts
In simultaneous-move games, players attempt to predict what their rivals will play and act accordingly.
A Nash equilibrium occurs when players’ expectations are are consistent with their opponents’ actions.
A Rationalizable strategy is a best response to some profile of one’s opponents’ strategies.
Nash Rationalizable.
Behavioral assumptions
Players use proper subjective probability distributions in analyzing uncertain events,
Players are expected utility maximizers,
The rules and structure of the game are common knowledge.
Rationalizability
A strategy profile for player is dominated if there exists another strategy that is better regardless of what other players do.
Given a profile of strategies of player ’s opponents, , a strategy for player is a best response if it is better than any other strategy given .
Let denote the set of player ’s strategies.
We say is a level–1 rational strategy for player if such that is a best response. Let denote the set of such strategies.
We say is a level–2 rational strategy for player if such that is a best response.
And so on…
I. 2x2 Game of Complete Information
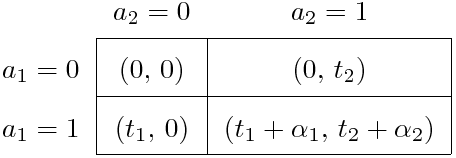
Standard 2x2 normal-form game.
Given a sample of market structures (entry decisions) in independent markets.
We want to learn about the joint distribution of as well as the parameters and .
Assume that .
I. Level–1 rationality
If , then is a dominant strategy for player 1.
If , , then is a dominant strategy.
If , then both and are level–1 rational. They are best responses, respectively, when player 2 plays 1 or 0.
Similarly for player 2.
I. Level–1 Rationality: Predictions
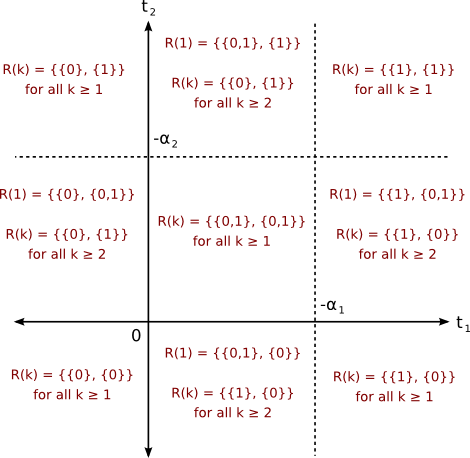
- In the middle region on the right side, note that player 2 does not rationally consider that player 1 would never play 0 in level–1 rationality. This illustrates the sequential nature of rationality.
I. Level–2 rationality
Consider the region .
Player 2 believes player 1 will play with probability 1.
is a best response.
We can eliminate at level 2.
for .
I. Inference
- Suppose we know the outcome probabilities .
- Object of interest: .
- is the joint distribution of .
- Level–1 rationality implies the following restrictions on :
- The identified set is the set of all which satisfy these inequalities.
- The model point identifies if is a singleton.
II. 2x2 Game of Incomplete Information
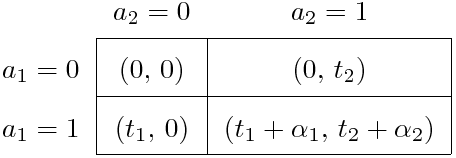
Now assume that and are private information.
Common prior assumption on the joint distribution of .
Players have beliefs about their opponents’ actions, conditional on their own type:
As before, assume that for .
The expected payoffs are now:
II. Rationality
Consider only threshold strategies: for .
Beliefs are thus probability distributions for given (which includes ): This assumption effectively reduces the space of possible strategies to .
The concept of rationality now has to account for level- rational beliefs. Beliefs are not required to be “correct.” Compare this with BNE beliefs, where all players know them to be correct.
Level- rationalizable beliefs assign zero probability to strictly dominated strategies by player .
A strategy by player is level- rationalizable if it is a best response given level- rationalizable beliefs:
- The support of by applying iterated elimination of dominated strategies.
II. Level–1 rationality
For any belief function, the following must hold eventwise:
All other decision rules are strictly dominated for all possible beliefs.
The above inequalities imply or simply, .
This is the set of level–1 rationalizable strategies.
II. Level–2 rationality
Level 2 rationalizable beliefs:
assign zero probability to strictly dominated strategies ,
satisfy and .
Level 2 rationalizable strategies are:
level 1 rationalizable (i.e., ),
best responses given level–2 rational beliefs.
A strategy is level–2 rationalizable if
II. Level-k rationality
We can summarize the set of level- rationalizable strategies in the class of threshold strategies as follows:
For and ,
For and ,
Note that for any a.s.
Any level- rational player is also level- rational for any .
If there is a unique BNE , then
II. A Parametric Model
Let for where is observable to the researcher but is not. We wish to estimate .
For simplicity, and are independent and .
Player knows and believes .
We now proceeds as follows:
Develop an objective function which can be used to construct the identified set ,
Discuss identification of ,
Provide sufficient conditions for point identification.
II. Iterative Construction of Beliefs
We no longer restrict ourselves to threshold strategies.
Let be the information set of both players as well as the econometrician (i.e., the regressors).
We iteratively construct bounds on the beliefs :
Initialize and .
Then for each and each :
It follows that
II. Iterative Belief Construction Example
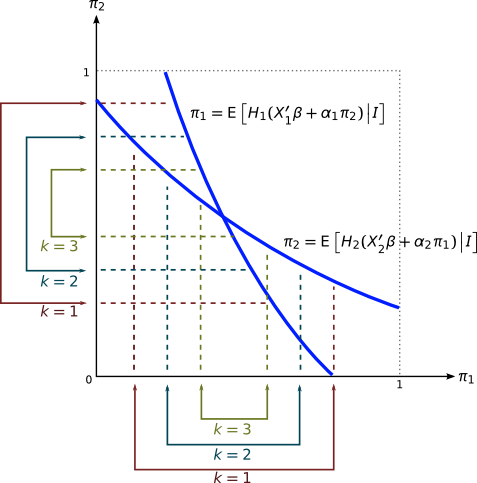
II. Finding the Identified Set
Player is -rational if and only if
We can use this relationship as a basis for inference.
Given some , let and let :
Then define
II. Finding the Identified Set
We can use the previous expression to construct an objective function.
For some positive definite matrix ,
By construction, for all .
Given a random sample, we can use set inference methods to construct an estimator for .
As compared with Nash equilibrium, we do not have to solve a fixed point problem. The iterative restrictions imposed by rationality are computationally simple by comparison.
For any , is guaranteed to contain the BNE.
II. Inference on the Rationality Level
A sample can also inform us about the level of rationality .
Suppose all players are at most -rational.
The level- bounds should hold a.e. but the level- bounds may be violated.
We can proceed as follows:
Construct ,
Define ,
for but if .
Thus, if and we can reject .
II. Point Identification Under Level–1 Rationality
Suppose players are level–1 rational.
Suppose has full rank for . Let and denote, respectively, regressors with bounded and unbounded support and let and be the corresponding coefficients. Finally, define .
is identified if for each , there is a continuous with nonzero and unbounded support conditional on such that for any , and , there exists such that for all and .
II. Point Identification Under Level–1 Rationality
is identified if for any with ,
If the above two properties hold, and for any , there exists such that whenever , then the identified set for is .
III. First Price IPV Auction
Many symmetric, risk-neutral potential buyers.
Bids are made simultaneously for a single good.
Focus on independent private values (IPV) .
Object of interest: , the distribution of private values.
is common knowledge among all players; has support .
For simplicity, assume the reserve price is .
III. Interim Rationality
Point identification in BNE case established by Guerre, Perrigne and Vuong (2000).
Here, the equilibrium assumption is relaxed following Battigalli and Siniscalchi (2003).
Buyers under interim rationality:
rational,
expected utility maximizers,
strategically sophisticated to a particular degree ,
have beliefs that may or may not be “correct.”
III. Assumptions on Beliefs
Bidders expect that any positive bid will win with positive probability:
No player will bid higher than ,
Each bidder with will submit a strictly positive bid.
The number of potential bidders equals the number of actual bidders a.s.
Beliefs only assign positive probability to increasing bidding functions.
The space of all such functions is
III. Level–1 Rationality
Player ’s problem is
Here, denotes player ’s beliefs about .
Level–1 rational bid satisfy
Any bid show any bid below is level–1 rational (and thus we cannot bound bids from below).
III. Level–2 Rationality
The worst case scenario for player is that for all ,
Expected utility in this case is
is thus a lower bound over all beliefs. The upper bound is .
Thus, rational bids must satisfy or
III. Level-k Rationality
Proceeding recursively, one can show that at level-, where and
We know that for all . Furthermore, bidding below is rationalizable for all .
That is, the predictive power of -rationality in this model is significantly less than that of BNE.
III. Identification with Level–1 Rationality
Suppose lies in the space of log-concave, absolutely continuous distribution functions on .
We are given a sample of auctions and want to recover .
The model predicts that level–1 rational bids satisfy for all .
The ’s are observed but the ’s are not, but we can still bound :
Empirical strategy: bound the distribution of valuations using the empirical distribution of bids.
III. Identification with Level-k Rationality
Continue for using the bounds derived above.
We can derive an objective function as before:
Conclusion
Look at identification power of Nash equilibrium by comparing to level- rationality.
Derivation of identified set and corresponding objective functions for conducting inference.
Three cases:
2x2 game of complete information,
2x2 game of incomplete information,
first price auction.