Graham, Imbens, and Ridder (2006)
These slides are based on the working paper “Complementarity and Aggregate Implications of Assortative Matching” by Bryan S. Graham, Guido W. Imbens, and Geert Ridder, May 14, 2006.
Presentation by Jason Blevins, Duke Applied Microeconometrics Reading Group, March 6, 2007.
Basic Model
Reallocation of an indivisible input across firms.
Aggregate stock of input is fixed.
Firm output may be monotone, but at different rates.
Cannot simultaneously increase input level for all firms.
Reallocations
What is the effect on average output of input reallocations?
Marginal distribution of reallocated input remains unchanged.
Average output may change if production technology is nonseparable.
Examples
Teacher reallocation across classrooms of varying mean student ability.
Assignment mechanisms for college roommates in the presence of social interactions.
Effects of spousal sorting on child education.
Estimation
Nonparametrically estimate production function, CDFs of inputs, and quantile functions.
Average over distribution of inputs under new assignment rule.
Comparison
Allow for continuous treatment (rather than binary or discrete).
Assignment policies do not change the marginal distribution of the input in the population.
In treatment effect literature, assignment of treatment is not restricted by the treatment of other units.
Focus on redistributions under specific assignment rules, not optimal assignment rules.
Contributions
Develop a framework for estimating outcomes under correlated matching.
Derive an estimator for average output under correlated matching.
Except for perfect positive and negative rank correlation, the estimator has a parametric rate of convergence.
Derive the asymptotic properties of the estimator in all cases.
Model
output associated with input level for firm .
Interested in reallocating input across firms.
Hold marginal distribution of fixed.
Observed firm characteristics , .
Notes:
Holding the marginal distribution of fixed is appropriate for situations where the input is indivisible (e.g., Teachers, Managers) and when the aggregate stock of the input is hard to augment.
is a scalar because the paper focuses on rank ordered matching. There is no clear natural ordering for vector valued covariates.
Identifying Assumption
Unconfoundedness/Exogeneity:
Conditional on firm characteristics , the assignment of is exogenous.
Example: . Then, and consequently, .
That is, the average output we would see if all firms were assigned equals the average output among firms that actually have . The distribution of potential outcomes must be the same in the subpopulation of firms that were assigned as that in the overall population. This is the analogous assumption to that of the binary treatment effect model of Rosenbaum and Rubin (1983).
- In general, this only has to hold within subpopulations.
Production Function
Define the production function
denotes average output associated with input levels .
Under the unconfoundedness assumption,
Unconfoundedness implies that among firms with identical and , the (counterfactual) average output of firms if we assigned to all firms is equal to the actual average output of firms that are in fact assigned .
Quantities of Interest
Treatment effect literature has mostly looked at estimating
With continuous inputs, we may want to estimate or
This paper is concerned with policies that redistribute an input following a rule based on .
What is the average output under such a policy?
Positive Matching
Among units with the same realization of , those with the highest values of receive the highest values of .
denotes the conditional CDF of given .
is the quantile of order of the conditional distribution of given .
We would expect this redistribution to perform well if there is complementarity between and .
is a conditional quantile function.
Graphical Example
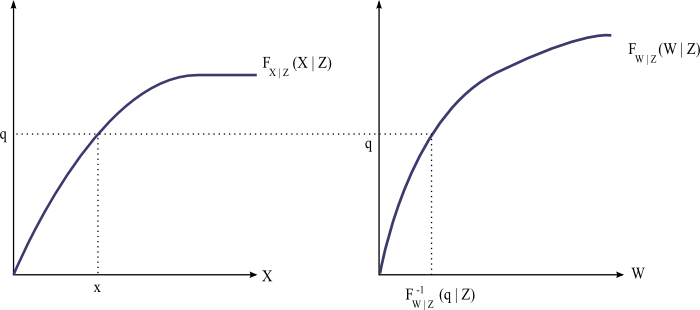
Interpretation
Thus, takes a unit’s position in the distribution of and assigns to it a value of corresponding to that quantile.
Consider instead the population-wide redistribution
Total effect is hard to interpret: complementarity or substitutability between and is mixed with correlation between and .
The authors focus on redistributions within subpopulations defined by because they reflect solely the complementarity or substitutability between and . Population-wide redistributions confound these effects by altering the joint distribution of and .
However, there is a distinction to be made between what redistribution might help us learn about complementarity and which might be optimal socially.
An Example
- : teacher quality.
- : mean beginning-of-year achievement.
- : fraction of class that is female.
- Suppose achievement varies with gender ( and correlated).
- Positive assortative matching: high-quality teachers assigned to high-achievement classrooms.
- Alters joint distributions of and as well as and .
An Example
- This also tends to assign good teachers to classrooms with a large fraction of female students.
- Subsequent increases in achievement may reflect complementarity between and .
- May also reflect how changes in teacher quality changes with gender.
- Conditional on gender, there may be no complementarity at all!
- Thus, focusing on redistributions across classrooms with similar gender mixes allows us to learn about complementarity.
Negative Matching
Example: assign best teachers to low-achievement classrooms.
Estimation
Note that although the model was developed for subpopulations, only population-wide estimators are presented.
We can estimate and only at nonparametric rates.
These estimators follow the analogy principle.
Status quo:
For others, need , , , and .
Estimation of g
Nonparametric Kernel estimation of the production function .
Series estimators could also be used.
Kernel with , bandwidth , and .
Support problems: we are trying to learn about a counterfactual allocation that may involve areas of the support for which we have few observations to estimate .
Estimation of CDFs
- Use empirical CDFs:
- Quantile function :
Estimation
- Estimate and by analogy:
Note that here we are averaging over both and .
The rate of convergence of and is slower than the parametric rate.
Loosely speaking, this is because we estimate a nonparametric function with more parameters than we then average over.
Correlated Matching
We have four focal allocations:
Perfect positive assortative matching,
Perfect negative assortative matching,
The status quo,
Random matching.
Random matching occurs when and are independently assigned within subpopulations.
Consider a subset of the set of all feasible allocations.
Two-parameter subset which has the above as special cases.
Traces paths between the four focal allocations.
Correlated Matching
controls nearness to the status quo (at 1).
controls nearness to perfect negative or positive allocative matching.
Focal allocations
Correlated Matching
Status quo allocation:
Random matching allocation:
Normal Copula
- Redefine matching allocations using a truncated bivariate standard Normal copula:
- The possible joint CDFs in this class are parametrized by
Normal Copula
Marginal CDFs: , .
Special case: independent and when .
Joint PDF:
Correlated Matching
- denotes distance from the status quo.
Estimation
- Analog estimator for :
Under random matching (), the densities on the right hand side cancel out, leaving .
For , we have the convex combination
This is linear in the nonparametric regression function and nonlinear in the empirical CDFs of and .
The authors claim that under certain conditions, this estimator is consistent and asymptotically normal, but the proofs are omitted in the latest available version (May 2006).
Application
Effects of parents’ education on education of child.
Data: 10,272 children from the National Longitudinal Survey of Youth (NLSY).
Simple model with three variables:
Mother’s education
Father’s education
Child’s education
Summary Statistics
Variable | Mean | Std. dev. |
---|---|---|
Ed. child | 13.06 | 2.38 |
Ed. mother | 11.20 | 2.87 |
Ed. father | 11.20 | 3.64 |
Regression
Variable | Coefficient | Std. Err. |
---|---|---|
Constant | 11.2700 | 0.1900 |
Ed. mother | -0.0410 | 0.0360 |
Ed. father | -0.0770 | 0.0290 |
Ed. mother2 | 0.0110 | 0.0023 |
Ed. father2 | 0.0110 | 0.0015 |
Ed. mother × Ed. father | 0.0014 | 0.0029 |
Nonlinearity of the relationship suggests that education of the child might be sensitive to reallocations.
Inspection of the data reveal that there is an asymmetry: it is better to have a mother with high education and a father with low education than vice-versa. The interaction term here doesn’t capture that.
Estimates
-0.99 | 11.5 | .069 |
-0.80 | 11.7 | .048 |
-0.60 | 11.9 | .040 |
-0.40 | 12.1 | .037 |
-0.20 | 12.4 | .034 |
0.00 | 12.6 | .033 |
0.20 | 12.8 | .031 |
0.40 | 12.9 | .030 |
0.60 | 13.0 | .029 |
0.80 | 13.0 | .029 |
0.99 | 13.1 | .039 |