Leveraging Uniformization and Sparsity for Computation of Continuous Time Dynamic Discrete Choice Games
Jason R. Blevins.
The Ohio State University, Department of Economics
Working Paper.
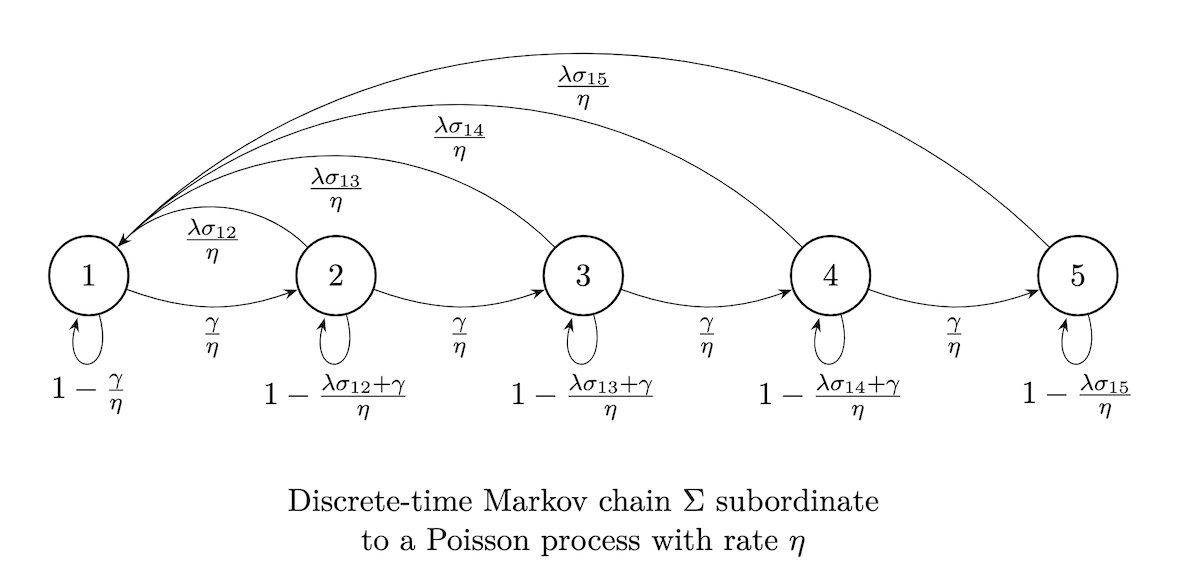
Availability:
- Working paper | arXiv (updated July 20, 2024)
Abstract. Continuous-time formulations of dynamic discrete choice games offer notable computational advantages, particularly in modeling strategic interactions in oligopolistic markets. This paper extends these benefits by addressing computational challenges in order to improve model solution and estimation. We first establish new results on the rates of convergence of the value iteration, policy evaluation, and relative value iteration operators in the model, holding fixed player beliefs. Next, we introduce a new representation of the value function in the model based on uniformization—a technique used in the analysis of continuous time Markov chains—which allows us to draw a direct analogy to discrete time models. Furthermore, we show that uniformization also leads to a stable method to compute the matrix exponential, an operator appearing in the model’s log likelihood function when only discrete time “snapshot” data are available. We also develop a new algorithm that concurrently computes the matrix exponential and its derivatives with respect to model parameters, enhancing computational efficiency. By leveraging the inherent sparsity of the model’s intensity matrix, combined with sparse matrix techniques and precomputed addresses, we show how to significantly speed up computations. These strategies allow researchers to estimate more sophisticated and realistic models of strategic interactions and policy impacts in empirical industrial organization.
Keywords: Continuous time, Markov decision processes, dynamic discrete choice, dynamic stochastic games, uniformization, matrix exponential, sparse matrices, computational methods, numerical methods.
JEL Classification: C63, C73, L13.